How generative AI and AI agents are impacting the market research playbook
By Andrew Littlefield●6 min. read●May 14, 2025
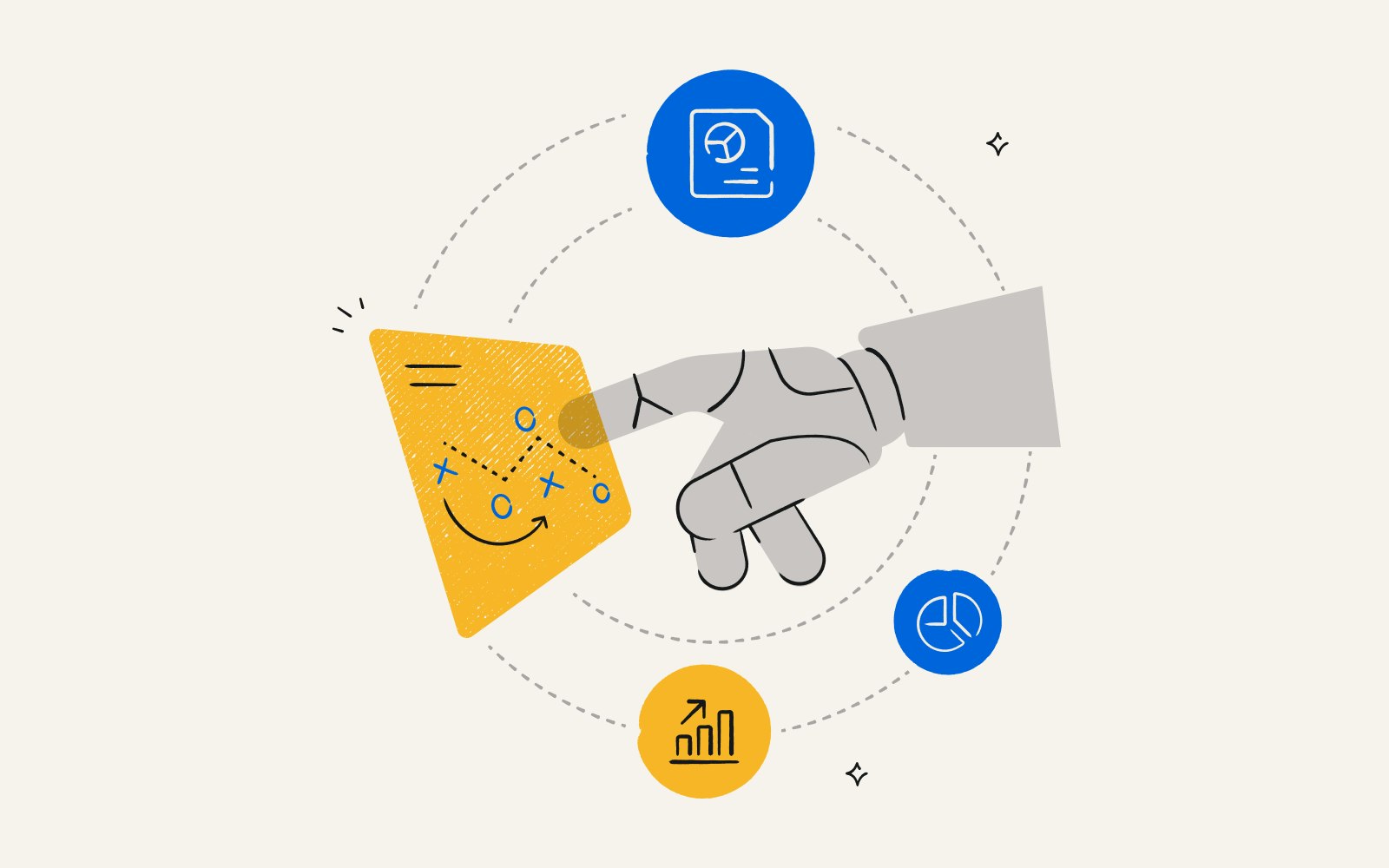
Market research has always been a game of scale.
Getting deep insights from participants (especially in qualitative studies) has historically been time-consuming, expensive, and limited to small sample sizes. It’s easy to survey 1,000 people if you're asking yes or no questions. It’s much harder when you want open-ended responses and thoughtful answers. Small teams are often left making decisions without the hard data to back it up.
“Many organizations say they’re data-driven,” says Dr. Stefano Puntoni, Sebastian S. Kresge Professor of Marketing at The Wharton School. “But most decisions are still made based on habit, gut feelings, or whatever the competition did yesterday.”
That’s starting to change.
Generative AI and agent-based tools are giving researchers new ways to scale open-ended insights, test ideas at speed, and even build “synthetic” versions of real customers to pressure-test decisions. But for all its promised benefits, this new technology comes with some major challenges and drawbacks as well.
It’s time to break down how generative AI is reshaping the market research playbook: what it unlocks, where it falls short, and what to watch for next.
4 ways generative AI is being used in research today
Generative AI is beginning to affect research workflows in a few different ways. These tools have the potential to speed up market research and generate more insights, but you should also account for their weaknesses.
1. Survey creation and refinement
Large language models (LLMs) are already being used to draft, translate, and refine survey questions. They can A/B test question phrasings, assist with translation, and adapt tone or complexity for different audiences. Tools like Outset.ai and Meaningful are baking these capabilities into streamlined workflows.
But automating survey creation comes with risk. LLMs are built to be helpful — sometimes too helpful.
“There’s a real danger that AI-generated survey questions reinforce confirmation bias,” said Dr. Puntoni. “Models like ChatGPT tend to agree with the user and validate their assumptions. If a researcher already has a bias, AI can amplify it, not challenge it.”
Researchers in AI call this quality sycophancy.
2. Qualitative analysis and theme generation
One of AI’s biggest breakthroughs for researchers is in summarizing open-ended responses at scale.
“Doing a qualitative study with 1,000 participants used to be impossible,” Dr. Puntoni said. “Now it’s feasible, even for small teams.”
LLMs excel at pattern recognition and language summarization, which makes them particularly adept at surfacing common themes from long-form responses. Researchers can extract insights, compare sentiment across demographics, or find recurring language.
That said, these models still hallucinate (meaning they make stuff up). LLMs sometimes invent insights or generalize too broadly.
“LLMs aren’t optimized to tell you the truth,” said Dr. Puntoni. “They’re optimized to sound correct. That makes it very easy for users to trust what feels like confident output, even when it’s wrong.”
This is where human review remains critical. AI can surface patterns, but only human researchers can validate whether those patterns are meaningful or misleading.
3. Real-time chatbot moderators and virtual interviews
One potentially promising application of AI in research is the use of autonomous agents to run interviews or moderate focus groups in real time.
These agents can (theoretically) be scripted to follow interview guides, adapt to user responses, and conduct dozens (or thousands) of interviews simultaneously. Unlike human moderators, they never get tired, and they log everything. Combined with automated analysis, automated moderation enables the kind of scale and repeatability that qualitative research has historically lacked.
“It blurs the line between qualitative and quantitative data,” said Dr. Puntoni. “You get the open-ended richness of interviews, but at a scale that starts to resemble a survey.”
While this sounds like a great use case, keep in mind the technology is still new and relatively untested. There’s also evidence that humans prefer human customer service over chatbots, which could mean chatbot-led interviews are skewed. Research teams are still on the hook to verify results.
4. Digital clones
Every marketer knows about buyer personas. They typically live in some slide deck, buried in your marketing strategy, outlining the different “personalities” of your target customer. But what if that buyer persona came to life?
“Digital clones are LLMs trained on your actual customers,” says Dr. Puntoni, “Market researchers can now create a buyer persona, and actually talk to it.”
Digital clones or “synthetic users” sound like science fiction, but they present big potential for research professionals — if they work, that is. Imagine not being limited by small sample sizes. Tools like SyntheticUsers or Evidenza allow researchers to simulate hard-to-reach users, like procurement leads or CFOs, and explore how they might respond to different products or messaging.
“If you’re looking to survey people who know about Coca-Cola or Nike, sure, no problem, just walk down the street and ask anyone,” says Dr. Puntoni. “But if you’re a startup trying to research chief HR officers or senior consultants, you’ll struggle to find five people willing to participate. Now you can create synthetic approximations of niche customer profiles.”
This approach isn’t perfect, but it’s often better than nothing, especially when real-user access is limited.
The other innovation involving digital clones is how these surveys are tested. Instead of piloting a survey with 50 real people, researchers can now run test flows with synthetic participants — digital personas that simulate real behavior. These synthetic runs can potentially identify survey drop-off points or highlight unclear questions before researchers spend money to get human participants.
The benefits of using gen AI for market research
What should excite market research professionals is how AI can level the playing field for small firms. Research has historically been expensive and time-consuming — something only big brands or specialized research firms could conceivably make part of their marketing playbook. But gen AI is starting to remove some of these barriers to entry, giving smaller companies capabilities they’ve never had before.
Potential benefits of generative AI for market research:
Speed. Drafting surveys, moderating sessions, translating materials, and summarizing responses can now take hours instead of weeks.
Scale. AI allows small teams to run large, complex studies that previously required agencies or large internal departments.
Cost-efficiency. Synthetic participants and automated moderation can cut down on panel costs, staffing, and vendor fees.
Accessibility. AI tools may lower the barrier to entry for small research teams that couldn’t previously justify large studies.
The challenges of gen AI for market research
AI might unlock new capabilities but it also introduces new risks. In April 2025, OpenAI CEO Sam Altman was forced to roll back changes to the company’s latest ChatGPT model.
“The last couple of GPT-4o updates have made the personality too sycophant-y and annoying (even though there are some very good parts of it), and we are working on fixes asap, some today and some this week,” Altman said via X.
As smart as they may seem, LLMs are still machines, and, like all machines, they’re prone to feedback loops and bias — exactly the kind of thing market research professionals seek to avoid.
Weaknesses of generative AI in market research:
Quality and representativeness. Synthetic personas are only as good as the data they’re trained on. If your source data is flawed or incomplete, the insights will be too.
Hallucination and overconfidence. LLMs don’t tell you what’s true — they tell you what sounds true. That makes them great at summarizing, but in need of careful human review to ensure accuracy.
Bias amplification. Generative AI has the potential to double down on human prejudices. “If you already have confirmation bias,” said Dr. Puntoni, “AI will put that on steroids.”
Ethical concerns. Using digital clones or simulated interviews raises questions about transparency, consent, and whether participants and moderators (real or synthetic) are being treated responsibly.
Over-reliance on LLMs. Perhaps the biggest risk of researchers using AI is forgetting that AI is a tool, not a replacement. “We need to make sure AI doesn’t substitute for critical thinking by a human expert,” said Dr. Puntoni. “That’s true not just for marketers, but all professions looking to use these tools.”
Increased risk of fraud. The ease of generating fake but realistic responses with AI also makes it easier for bad actors to submit fraudulent research data, especially in incentivized studies. Without robust validation methods, researchers risk basing decisions based on responses from bots or individuals gaming the system with AI-generated answers.
In short: AI can make research easier, but it doesn’t make researchers obsolete. Human expertise and judgement are still essential when evaluating human opinions, preferences, and motivators.
Key takeaways
Generative AI is already transforming how surveys are written, interviews are conducted, and open-ended responses are analyzed.
AI can theoretically allow smaller research teams to run bigger, faster, and more complex studies — including those that simulate hard-to-reach user types.
LLMs come with risks: sycophancy, hallucinations, confirmation bias, overconfidence, and ethical ambiguity, which all require human involvement and oversight to mitigate.
AI can introduce heightened risks for market research, including fraudulent responses, which makes it essential to use strong validation measures and tools with fraud prevention options — especially for incentivized studies.
The most effective researchers treat AI as a partner instead of a shortcut.
FAQs
Updated May 14, 2025